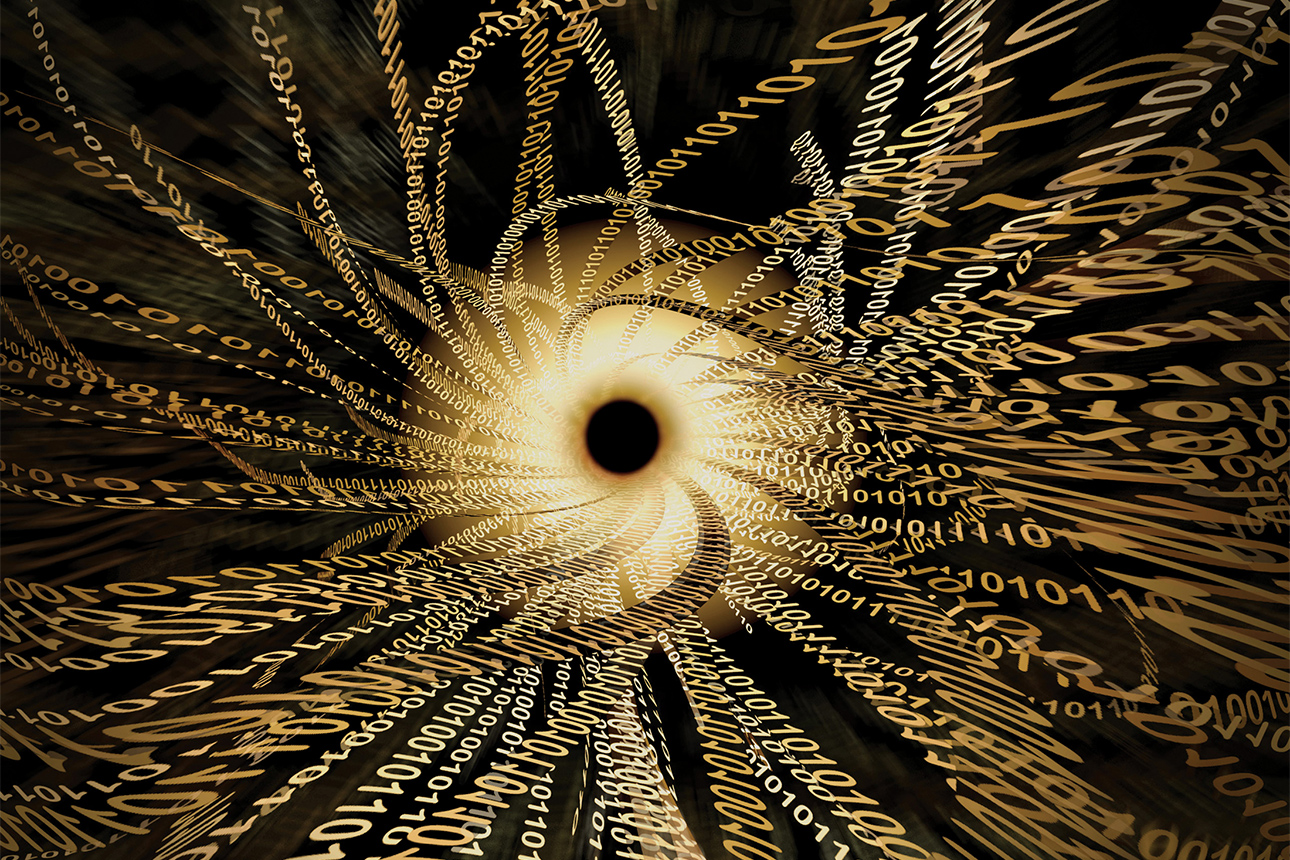
Image courtesy of Jean Francois Podevin/theispot.com
The idea that many businesses rely heavily on data to produce or market goods and services is not new.1 Indeed, even in 2018, four of the six top companies in market valuation — Amazon, Alphabet, Facebook, and Alibaba2 — based their business models on the use of data to optimize advertising. However, data differs greatly from traditional factors of production, such as capital and labor. For instance, to achieve scale, companies need data about large numbers of customers — especially when algorithms are used in advertising and other revenue-generating models. Given that scale, data interacts with personal privacy — even national security — in ways that other factors of production do not. These special attributes of data hinder its efficient and transparent trade in data markets, keep it in closed silos despite its digital nature, and often stop organizations from maximizing its value.
But the conception of big data as a silo managed by single entities is giving way to the notion of shared data. We are interested specifically in data exchanges — shared platforms where data is gathered and curated from many different sources (all the individuals and organizations that voluntarily share it), allowing third parties to gain insights from it. As those insights start to move freely, securely, and confidentially in the market, they will greatly enhance data-based value generation. But for that potential to be realized, managers must become familiar with the unique characteristics of data and with how data exchanges can capitalize on them while mitigating threats.
What Makes Data Unique
Appreciating the full potential of shared data starts with understanding how data is unlike other factors of production:
Data is non-fungible. Distinct units of data can be used differently by the same company. For example, when a business receives, say, $1 of investment, it is irrelevant which specific U.S. dollar of the many in circulation the business receives and whether the investor pays this dollar as one note, four quarters, or 100 cents. That’s because capital is interchangeable. However, should a company receive one unit (say, a megabyte) of data to develop a specific algorithm, not all units of data (health-related data, financial data, geolocation data, and so on) will serve the organization equally well in that effort.
Data is nonexclusive in its use. Two companies can use the same data at the same time.3 This is not the case with, say, capital (generally speaking, a dollar can be invested in only one business at a time) or labor (a person’s hour of work is particular to one work setting).
Data rapidly becomes obsolete. Data can change on a daily or even hourly basis, such that newer data is often more valuable. That’s not the case for all data (such as a person’s birth date, which never changes), but it certainly is for health-related, financial, and geolocation data. Other factors of production also can become obsolete. But it takes years for capital to depreciate, for example, and workers whose skills become redundant can be retrained. Data’s value typically declines much more quickly — often forever.
Data generates value mainly in large volumes. Small amounts of data can occasionally be valuable, but not for conducting analytics, training an algorithm, or scaling a business to many customers. In most business contexts, only the aggregation of big data has substantial value.
Data is often created when two or more instances of use interact, not in isolation. Think of how Amazon, Facebook, Google, and Alibaba cocreate — with their platforms’ users — the data that reveals valuable insight about user behaviors and preferences.
Individuals have rights over their raw data. It is illegal to sell, share, exchange, or trade a person’s data without his or her informed consent. A worker does have some rights of consent (for example, refusing to engage in criminal activity for an employer), but those rights are limited. And people control their own capital investments. However, personal data can be (and has been) used without individuals even noticing it, making consent concerns qualitatively different for data than for other factors of production.
Current Obstacles to Sharing Data
Data, unlike capital and labor, does not yet have a transparent, global market that permits its mobility from individuals to organizations and between organizations. Therefore, companies and platforms tend either to work only with the data that individuals (their clients or users) have generated within the organization4 or to purchase data from aggregators in an opaque manner, preventing individuals from participating in, influencing, and directly benefiting from the trade. Consider Google’s Nightingale Project,5 whereby Google has purchased health care data held by Ascension, the second-largest U.S. health care provider, without patients having any say in the deal or directly benefiting from it. (The Nightingale Project is currently under investigation by the U.S. Department of Health and Human Services.6)
Because data is non-fungible, it is difficult for an individual to sell the rights to his or her data directly to an organization. The prospective buyer would need to assess the value of the (unstructured) data before purchasing it — a feat that is technically possible but complex and associated with high costs. In addition, most organizations are interested only in purchasing data about many people, because only high volumes of diverse data typically yield worthwhile insights, so individuals have little or no bargaining power. Also, without the informed consent of the individual, it is illegal to offer third parties access to personal data that is not aggregated or sufficiently anonymized, making the purchase process a difficult, multistage negotiation.
The fact that data — particularly data that users cocreate when interacting with online platforms and services — is not transparently traded is economically rather inefficient. For one thing, the data cannot move to the companies for which it generates the most valuable insights. For another, there are no standard terms for data purchases — which impedes the efficient allocation of an organization’s resources. In contrast, capital and labor move freely in the open market to the companies where they can yield the highest returns (interest in the case of capital; wages and career growth in the case of labor).
How Data Exchanges Facilitate Data Sharing
Data exchanges are usually managed and controlled by a foundation, a private company, or a cooperative of users. They generate value by structuring, aggregating, and anonymizing the data that providers voluntarily share — and by allowing third parties to run algorithms on it. In turn, third parties either pay fees that are ultimately distributed among the data providers or, if the exchange is a cooperative of individuals and their data, they may choose to offer enhanced services in lieu of fees. Some data exchanges, such as OPAL7 and X-Road,8 use blockchain technology to enable a transparent governance structure that helps to reassure providers that they can safely share their data.
Data exchanges are popping up around the globe, with the U.S. as a leading hub, followed closely by Singapore, Australia, and Europe. Although data exchanges are new and no clear standard-bearer or market leader has emerged, the number of them seeking to become “the one” is rising. A simple internet search reveals dozens of them.
To better understand the value they can generate, consider this hypothetical scenario: A pharmaceutical company with a bright idea for a new venture, a suitable team, and sufficient capital seeks to generate a new algorithm for detecting a particular illness at an early stage. Before data exchanges existed, the company would have faced great hurdles in gathering or legally buying the large amount of specific data needed to train the algorithm. Nowadays, a data exchange that receives medical records voluntarily provided by patients has the data it needs for the algorithm — and it can receive (from the pharmaceutical company) fees or medical services that get distributed to the patients whose data has been used.
In this new world of data exchanges, data owners (in this case, patients) make their own data work for them in return for a fee or an enhanced service. Simultaneously, third parties (such as the pharmaceutical company) generate value that didn’t exist before the data exchange.
The non-fungible nature of data on an exchange is key. Given that third parties can run algorithms on the data only after it is deidentified, aggregated, and structured, the exchange knows specifically on which data the third parties must run their computer code and what that code computes.
In addition, because exchanges aggregate data from many different agents, they can provide the diversity and volume that algorithms require. And the exchanges — as long as the algorithms are properly audited — give third parties only “safe” answers that don’t reveal privacy-violating information from data providers. The pharma company, for example, would have access to data about enough patients of a particular type but could not link any specific medical record to a particular patient’s identity. That greatly simplifies the problem of obtaining individuals’ consent. Furthermore, because a data exchange aggregates the interests of many data owners, it is in a stronger position than individual data owners to assess prices and negotiate.
Moreover, the value of the data on data exchanges is typically transparent, allowing third parties to sell insights from the data at an adequate price and to distribute the resulting earnings among the original providers of the data. In the example above, patients can see when their data is being used to train an algorithm, as well as the amount the third party is paying to the exchange for running the algorithm on their data.
Data Exchanges in Practice
Real-world data exchanges include DSpark,9 Data Republic,10 Ocean Protocol,11 Dawex, and Enigma.12 Ocean Protocol, for example, gathers data from individuals, organizations in a range of industries, and even other data exchanges to benefit third parties and, ultimately, customers. For instance, driving data gathered from automakers might be used to help developers of software for autonomous vehicles; or data on employees’ workplace satisfaction, gathered from HR departments, might be used by companies to design better benefits packages and career-growth plans. Ocean Protocol, managed by a Singapore-based nonprofit foundation, is already working with companies such as Roche and Unilever. Data Republic, which uses a similar process for gathering and sharing data insights, is currently used by banks, airlines, and governments in Australia, New Zealand, the U.S., and Singapore.
The OPAL13 initiative is a proto-standard for data exchanges. OPAL’s purpose is to make a broad array of data available for inspection and analysis without violating personal data privacy, using three processes:
- The algorithm is moved to the data, such that raw data never leaves its repository and only “safe” answers are returned to the third party.
- The applied algorithms are open, such that they can be studied by experts who deem them to be safe.
- New-analysis technology14 allows the data to always be kept in an encrypted state, so that even the exchange cannot see the raw data.
Beyond commercial applications, data exchanges also are starting to be implemented for governmental and nonprofit use. An OPAL-style platform has recently been adopted by Eurostat for the exchange of all official European Union government data. And Estonia is using X-Road,15 a technological and organizational environment that enables a data exchange to securely move electronic health records, as well as data related to taxes, schooling, or land ownership.
The Macroeconomics of Data Exchanges
Data exchanges have the potential to be as positive for the economy as a well-trained labor force or newly found oil reserves. The difference is that the labor force and the oil would typically be used by only one company at a time, whereas data can be shared among many companies. Big data has already transformed the global economy; the impact of shared data via data exchanges might well have the same potential.16
For instance, data exchanges could cause current monopolies to confront new competitors. Today a young tech company will have difficulty competing with established giants because its lack of data prevents it from developing useful algorithms. As data scholars have noted, “During the last three decades the annual rate of new startups has fallen from 13% to less than 8% [and] the percentage of employment at firms with fewer than 100 workers has decreased by 5%.” Meanwhile, “the share of revenue of the top 5% of businesses has increased by 10%.”17 Data exchanges could reverse this trend, because startups and small and midsize enterprises could use capital to purchase data-based insights in the exchanges and then find themselves in a more competitive position than they are today.
Cooperatives Shift the Balance of Power
Data cooperatives allow individuals to get paid for the data they create and to exercise more pricing power than they would have on their own or in another type of data exchange. Examples include cooperatives of music artists, video producers, and gig workers. The income is not a subsidy, but rather the result of individual economic activity channeled through exchanges that aggregate the data of producers and workers, thereby turning individuals into data entrepreneurs. Market supply and demand determines the size of the “rent” that data owners can charge for their data. In some circumstances, that rent might substantially supplement a person’s income.
When 19th-century workers became aware of the value of their labor, many sought to form unions, collectively negotiate, exercise political lobbying, and bargain — from a stronger position — with the holders of capital. As people get economic returns on their data, many will develop a data consciousness, causing new data cooperatives to form.
TheGoodData, European Data Union, Data Workers Union, and The Data Union are established data cooperatives that aim to unite the data producers of the world. When data owners sell their data in regular, noncooperative exchanges, they indirectly reveal the data of other users, which depresses the price of data.18 That negative consequence can be avoided with coordinated selling of data by data cooperatives.19 Notably, existing credit unions have the means (access to millions of users who trust them with their money) and are in the legal position to become promoters and beneficiaries of data cooperatives. They would just need to start aggregating data rather than merely capital.20
Companies and platforms also might use data cooperatives to integrate their clients and users. By providing high-quality application programming interfaces, they could transfer data to a cooperative via a user-friendly system that new and existing clients would see as an added value — one for which the company or platform could charge. Such an idea would also align with the U.S.-based Business Roundtable’s recent shift toward a stakeholder-focused philosophy.21 A cooperative model could even be perceived as an extension of Germany’s social market economy, with its close cooperation between labor unions and businesses.
Next Steps for Managers
By purchasing insights from data exchanges, companies can generate value from data they don’t currently own. For example, a producer of fertilizer that is interested in developing products for certain crops can directly purchase data insights from exchanges that hold data from farmers and learn how their crops respond to different products.
Companies also can use the data they generate themselves to become data producers, not just users of data exchanges. That’s a revenue-generating opportunity. For instance, agricultural companies raising animals can provide images of healthy and ill animals that can be used by insurance and pharmaceutical companies to train image-recognition tools that detect animal diseases.
Deciding whether to embrace or avoid collaboration with data exchanges is especially important for managers whose companies already host and analyze data about clients or users. If businesses decide to avoid interactions with data exchanges, their clients or users might integrate themselves into a data cooperative, excluding the companies from any say in the deal. If managers instead decide to embrace collaboration with data exchanges, they’ll be able to influence the architecture and functioning of data exchanges — and propose incentive structures with which their companies feel comfortable.
Managers also will have to determine how to communicate about data exchanges with their clients and about the data cooperatives that emerge within their organizations. As early as possible, companies should define their public position about data cooperatives and, internally, identify who will value the data that the company is hosting.
In addition, businesses and platforms can offer their clients and users solutions for sharing the revenue generated from participation in data exchanges. Herein lies an opportunity for differentiation from competitors, and for a new value proposition to retain existing clients and attract new ones.
Business managers should thoughtfully examine how data exchanges will shape their strategy and the economy more broadly — and then act on their conclusions. This work will help companies migrate successfully to the era of shared data and shape the economic ecosystems that emerge from this new reality.
What Managers Need to Know About Data Exchanges
No comments:
Post a Comment